Generative Modelling on Big Medical Datasets
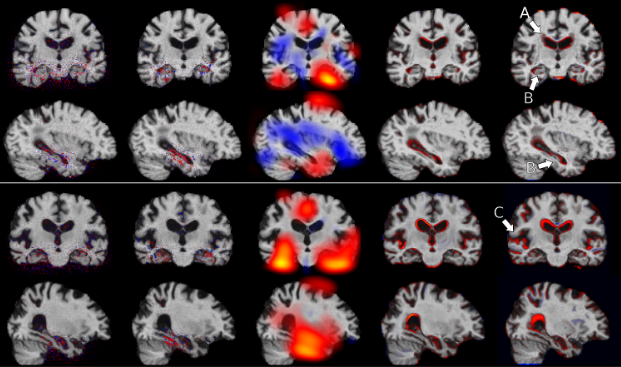
Large medical datasets are an invaluable asset for advancing clinical and machine learning research alike. Having realised this, many governments and organisations started collecting massive and incredibly detailed datasets of patients suffering from diseases as well as healthy people. Some examples of such datasets are the UK Biobank, the German National Cohort Study, or the Alzheimer’s Disease Neuroimaging Initiative. These dataset contain not only images, but vast amounts of extra information such as genetic markers and life-style choices. In parallel, machine learning technology, in particular the fields of generative modelling and causal inference have progressed to a point where analysing such datasets on a pixel/voxel level becomes feasible. This creates an unprecedented opportunity to discover physiological mechanisms, disease processes, and connections between imaging and non-imaging data. A focus of our group is to develop scalable probabilistic modelling and inference techniques to analyse such data.
Selected publications
Christian F Baumgartner, Lisa M Koch, Kerem Can Tezcan, Jia Xi Ang, Ender Konukoglu, Visual feature attribution using Wasserstein GANs, Proc. CVPR 2018