Meta-Learning for Diverse Medical Imaging Tasks
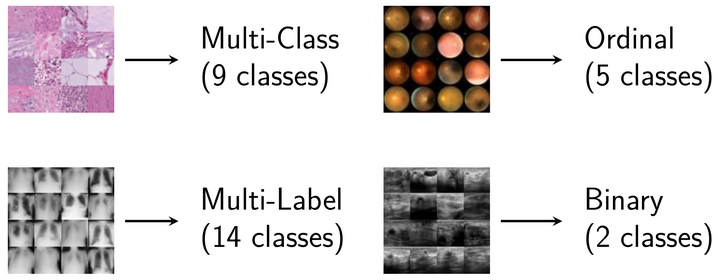
A major limitation of deep learning for medical applications is the scarcity of labelled data. Meta-learning, which leverages principles learned from previous tasks for new tasks, has the potential to mitigate this data scarcity. However, most meta-learning methods assume idealised settings with homogeneous task definitions. The most widely used family of meta-learning methods, those based on Model-Agnostic Meta-Learning (MAML), require a constant network architecture and therefore a fixed number of classes per classification task.
We take a first step in the direction of making meta-learning, and in particular MAML, suitable for more realistic medical problems by investigating different strategies for training and testing with a variable number of possible labels.
Publications
- Stefano Woerner, Christian F Baumgartner: Strategies for Meta-Learning with Diverse Tasks, MIDL 2022 Short Papers (2022)